Marketing mix modeling (MMM) vs. multi-touch attribution (MTA): Which is right for you?
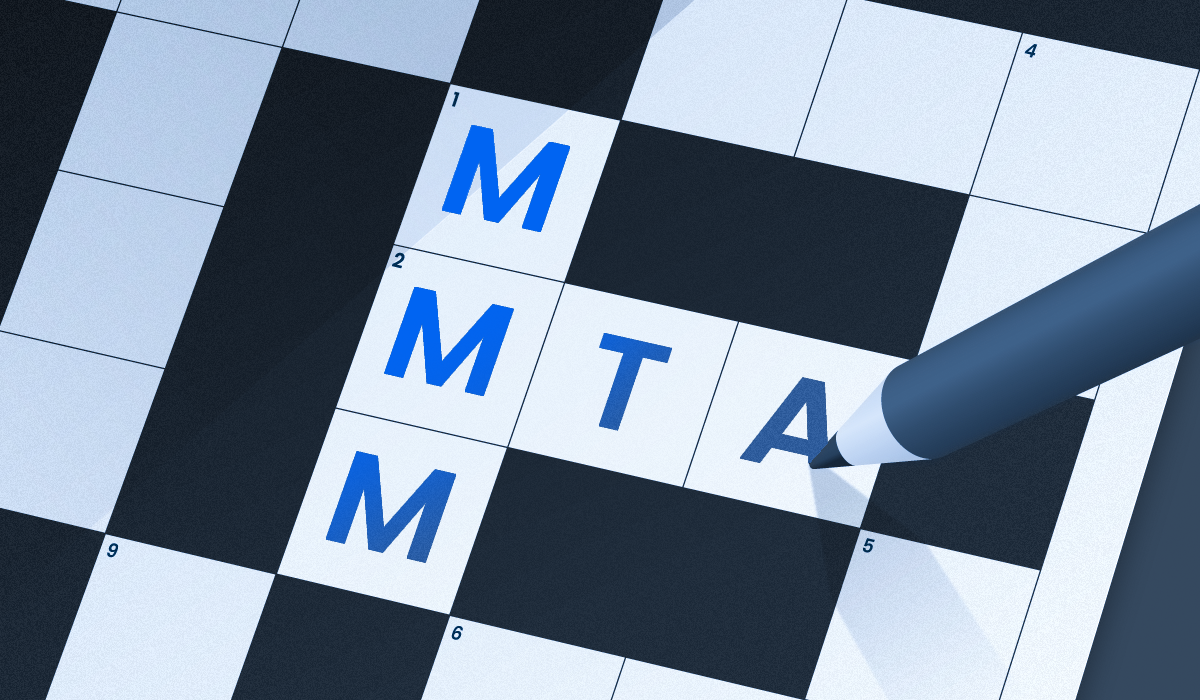
With so many channels available in the modern marketing world, marketers are always in search of powerful and sophisticated tools to pinpoint which mix of channels generate the most conversions and the biggest return. Marketing mix modeling (MMM) and multi-touch attribution (MTA) are two different approaches that can be used to understand the impact of marketing efforts on sales and other outcomes.
While both can uncover business insights, they also have some key differences that make each other more useful than the other depending on the circumstances. What are the advantages of the two approaches, and which is the best for maximizing marketing effectiveness? Read on to find out.
One of the main differences between the two approaches is their objectives. MTA sheds light on the impact of individual touchpoints on conversions and sales. It allows marketers to attribute credit for a sale or conversion to multiple touchpoints in the user journey, and to understand the relative importance of each touchpoint. MMM, on the other hand, is focused on understanding the overall impact of a company's marketing mix on its sales and other outcomes. It typically involves building a statistical model that incorporates data on marketing activities, such as ad spend and promotions, as well as data on sales and other outcomes.
Another key difference is the type of data that each approach uses. MTA relies on granular device-level data, which allows marketers to track individual users and their interactions with different touchpoints. MMM, on the other hand, typically uses aggregated data at the campaign or channel level, which can make it more difficult to see the impact of individual touchpoints on conversions and sales.
Yet, MTA and MMM do share a commonality: both approaches are not actual experiments, but observational models that are based on machine learning or statistical methodologies. To guarantee their reliability and accuracy, the results can be calibrated using lift studies.
The strongest advantage of MMM is that it is privacy-friendly. As described above, MMM uses aggregated campaign- or channel-level data rather than granular device-level data. This means that it can still provide valuable insights despite limited access to device-level data. For example, an MMM model could still be built using data on ad spend and touchpoints, as well as data on revenue and other conversions, even if there is no device-level data available.
Another advantage of MMM is that it can be used for both prediction and optimization. For prediction, the model can estimate the likely impact of different marketing strategies on revenue and other metrics. This comes in handy when developing future marketing strategies as it allows marketers to make more informed decisions about how to allocate their budgets and where to focus their efforts.
For optimization, the model can identify the most effective marketing channels that need to be scaled up. By running simulations and comparing the results of different marketing scenarios, marketers can use MMM to identify the marketing mix that will most probably lead to the best results. This can be useful for optimizing the performance of existing campaigns, as well as for planning future marketing efforts.
Although MMM has multiple notable advantages, it does have some potential drawbacks. One is that it may require additional data features, such as macroeconomic variables like GDP and market indexes, as well as internal information like scheduled product releases and promotions, to more accurately perform the analysis. This can be a challenge for marketers who may not have access to all of these types of data.
The greatest part about MTA is that it is more granular than MMM, allowing marketers to better understand the impact of individual touchpoints on conversions and sales. This makes it a better option for many marketers who are looking to optimize their marketing efforts and improve the effectiveness of their campaigns.
Another advantage of MTA is that it can be combined with incrementality measurement to draw causal inferences. Using techniques such as propensity score matching (PSM), marketers get to calculate a propensity score for each user based on device-level or user-level features and match the appropriate control and treatment groups. This minimizes the selection bias that may be present in basic observational models.
After determining the incrementality of the overall user journey, marketers can distribute the credits to each touchpoint using a weighting algorithm or other method. Combining MTA and incrementality measurement provides a more accurate and comprehensive view of the effectiveness of their marketing campaigns and helps with data-driven decision-making as well as campaign optimization.
MMM provides a top-down perspective of every aspect of a business and attempts to understand how each aspect contributes to a business outcome. MTA is a bottom-up approach, attempting to meticulously measure every consumer exposure to media and then attribute each exposure to an outcome.
Going back to our title – which one approach is right for you, the answer is neither. At Airbridge, we recommend using both MTA and MMM to get a full visibility of your marketing performance, rather than relying on just one, because they have competitive advantages at different stages of the marketing cycle. If you need more information to expedite your decision-making process, our MMM white paper can definitely be of help — go check it out!